For lifetime-lubricated components in the field of e-mobility, grease performance is a decisive factor that determines the performance and service life of an entire unit and ultimately the entire vehicle. Around half of all rolling bearing failures are due to a malfunction caused by inadequate lubrication or incorrect lubricant selection. This indicates that laboratory tests carried out in advance were not suitable for correctly predicting (simulating) the practical application or that the tests carried out and their results were misinterpreted.
Project start: Machine learning in lubricating grease evaluation
During the development of lubricating greases, numerous chemical-physical and mechanical-dynamic measurements and tests are carried out in order to determine the suitability for a planned application. Even today, the evaluation and interpretation of these measured values is still based on the experience of a few experts. Computer support for this process or even a computer simulation of real tribological systems running in boundary and mixed friction is not yet possible, apart from a few very scientific special cases. The diverse interactions between the surfaces, wear particles, lubricant and ambient medium in conjunction with the changes in the lubricant over time and non-linear system behavior cannot yet be simulated and predicted macroscopically. The reason for this is that the complex relationships cannot yet be adequately described mathematically/physically and extreme differences in size from atomic chemical processes to the macroscopic shape of the components have to be taken into account.
BMWK funds research projects
As of March 1, 2024, the BMWK is funding the DGMK project 871 "Use of machine learning in lubricating grease evaluation" as part of joint industrial research.
The aim of the project is to demonstrate the potential of machine learning in lubricant development. As a concrete application example, the extensive data of the predecessor project DGMK 820 on thickener degeneration as a result of thermal, catalytic, oxidative and mechanical stress will be analyzed using various ML methods and algorithms. In this way, the boundary conditions, advantages and limitations of this innovative approach can be made tangible for industrial companies.
Research Center
Mannheim University of Applied Sciences Tribology Competence Center
Network and cooperation
The project was initiated by members of the DGMK Lubricants Technical Committee and is being carried out in cooperation with experts from the FVA Forschungsvereinigung Antriebstechnik e.V. (Research Association for Drive Technology).
The project "Use of machine learning in lubricating grease evaluation" (funding code 01IF23227N) is funded by the Federal Ministry of Economics and Climate Protection as part of the "Industrial Collective Research" program based on a resolution of the German Bundestag.
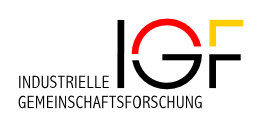
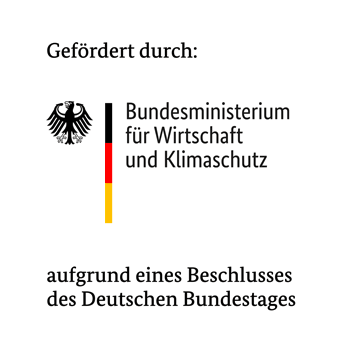